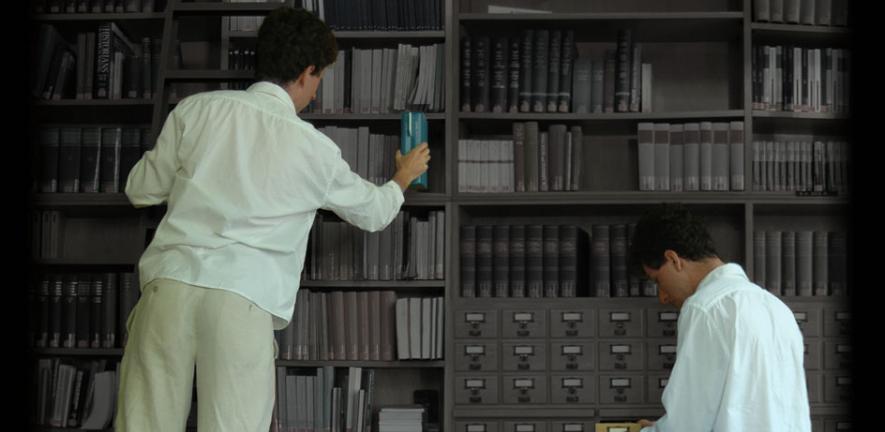
‘I raised to my lips a spoonful of the tea in which I had soaked a morsel of the cake. […] And suddenly the memory returns.’ À la Recherche du Temps Perdu, Marcel Proust.
‘I raised to my lips a spoonful of the tea in which I had soaked a morsel of the cake. […] And suddenly the memory returns.’ À la Recherche du Temps Perdu, Marcel Proust.
One particular challenge in memory research is to unfold the sequence of events happening at the level of nerve cells (neurons) that leads to the retrieval of a memory.
Recalling events from our past is a task we need to accomplish several times every day – even if the form it takes is usually not nearly as dramatic as Marcel Proust describes in his famous novel, in which a mere taste evokes memories long buried. For centuries, artists and philosophers have been intrigued by the process of memory storage and recall. More recently, psychologists, neuroscientists and cognitive scientists have begun to unravel some of the principles of ‘remembrance of things past’. And now, a branch of neuroscience that works on quantitative models of the nervous system – computational neuroscience – is also contributing to the quest.
Dr Máté Lengyel, at the Computational and Biological Learning Lab in the Department of Engineering, is borrowing ideas from machine learning to elucidate the principles of memory recall. One particular challenge in memory research is to unfold the sequence of events happening at the level of nerve cells (neurons) that leads to the retrieval of a memory. Not only is this a fundamental question in neuroscience, but it has also provided some of the finest examples of how collaboration between theoretical and experimental approaches can be especially fruitful for understanding the brain.
Attractor networks – attractive theories
A commonly held view in modern neuroscience is that most forms of memories are stored in the nervous system because of the changing way that connections (or synapses) are made between neurons – a phenomenon known as ‘synaptic plasticity’.
Once a memory trace has been laid down in a set of synapses it has to be recalled by neurons interacting with each other through these synapses. The first coherent picture about how this might happen was proposed by theorists who developed a specific class of neural network models called ‘attractor networks’. This theory has provided an elegant mathematical formulation of how a network of neurons gradually reconstructs a complete memory trace starting from only partial information – just as in Proust’s novel, the narrator recalls an entire scene starting from only the taste of cake soaked in tea.
Despite the success of attractor networks as a theoretical framework that guides many scientists’ thinking about the neural bases of memory storage and recall, there are several questions that the original theory is unable to address. Is it important just that a neuron is active or not, or is it the graded level of activation that bears information about the original memory trace? If we take it a stage further, do neurons only communicate through the average rate of the transient electrical impulses they emit, called spikes, or is it the precise timing of these impulses that are important?
It is well established, for instance, that the graded activity level of neurons and their spike timings play a central role in the functioning of the hippocampus, a brain structure in the medial temporal lobes that is crucial for an intact memory. Yet, traditionally, the theory of attractor networks assumes that memories are binary and based on the rate of spiking, and it has proved notoriously difficult to bridge this gap between theory and biological reality.
Bits and brains
Rather than following the more traditional path that starts from known biological properties of single neurons and synapses, and proceeds by analysing the emergent network behaviour they give rise to, Dr Lengyel is taking a different approach to understanding how memory processing is achieved by neuronal networks. This approach, pioneered by Professor David MacKay at the Cavendish Laboratory, first studies the task posed by memory recall as a special case of ‘statistical inference’, a mathematical theory forming the foundations of many of today’s most powerful machine learning applications. The core idea is that storing memories in the synapses that connect a set of neurons is formally equivalent to data compression – something we are all familiar with, for example, when storing music as an MP3 file on an audio player. Memory recall then becomes an act of ‘decompressing’ the information previously stored in these synapses.
The next step is to take the position of an engineer who needs to construct a device that achieves the highest possible performance given the constraints provided by biology. This allows one to attempt to predict the properties of neurons and synapses that would be optimal for retrieving memories. Using the mathematical analogy between memory storage in neural networks and data compression, it has become possible to address the question of how neurons could implement the optimal decompression algorithm for recalling memories if the memories are represented by the graded activities of neurons using precise spike timings.
With recent funding from the Wellcome Trust, Dr Lengyel, together with Professor Peter Dayan at the Gatsby Computational Neuroscience Unit, University College London, is pursuing this direction of research. They work closely with Dr Ole Paulsen at the University of Oxford, whose group conducts experiments to test the predictions of the model in the neural networks of the hippocampus, and has already successfully confirmed some of the precepts of the theory.
The aim is to understand how the changing connections between the brain’s neurons maximise the information that is stored, providing the brain with the ability to remember. The aim also is to show how different rates of neural spiking might represent the level of certainty that a memory being recalled is correct. Both of these issues are important if one wants to design a device that presents an optimal solution to the task of memory recall, and allows us to ask whether nature has designed our brains in such a way.
For more information, please contact the author Dr Máté Lengyel(ml468@cam.ac.uk) at the Computational and Biological Learning Lab in the Department of Engineering.
Minds and machines
The same mathematical and engineering principles that can be used to understand learning in the human brain can also be used to build artificial learning systems. This interplay between human and machine learning is the main research focus of the recently established Computational and Biological Learning Lab (CBL) at the Department of Engineering. CBL was founded in 2006 with the arrival of Professor Daniel Wolpert and Professor Zoubin Ghahramani in Cambridge, and has rapidly grown to include Dr Carl Rasmussen, Dr Máté Lengyel and over 20 PhD students and postdoctoral researchers. CBL is investigating the computational principles underlying human sensorimotor control, the design of computer algorithms that learn, adaptive reinforcement learning controllers, statistical theories of learning, and how networks of neurons can perform computations.
For more information, please contact Professor Zoubin Ghahramani(zoubin@eng.cam.ac.uk) or visit https://learning.eng.cam.ac.uk/Public/
This work is licensed under a Creative Commons Licence. If you use this content on your site please link back to this page.