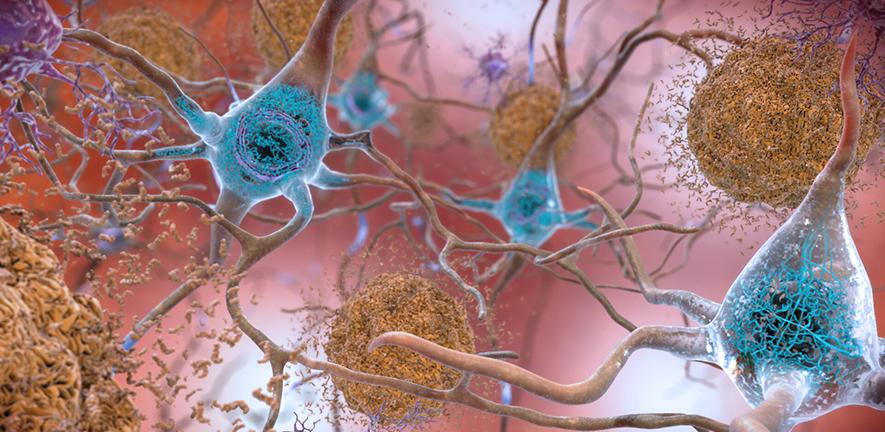
Study shows how to determine the elusive motions of proteins that remain disordered.
Study shows how to determine the elusive motions of proteins that remain disordered.
The constant motion of amyloid-beta is one of the reasons it’s been so difficult to target – it’s almost like trying to catch smoke in your hands
Michele Vendruscolo
Researchers from the University of Cambridge, Google Research and the University of Milan have used machine learning techniques to predict how proteins, particularly those implicated in neurological diseases, completely change their shapes in a matter of microseconds.
They found that when amyloid-beta, a key protein implicated in Alzheimer’s disease, adopts a collection of disordered shapes, it actually becomes less likely to stick together and form the toxic clusters which lead to the death of brain cells.
The results, reported in the journal Nature Computational Science, could aid in the future development of treatments for diseases involving disordered proteins, such as Alzheimer’s disease and Parkinson’s disease.
“We are used to thinking of proteins as molecules that fold into well-defined structures: finding out how this process happens has been a major research focus over the last 50 years,” said Professor Michele Vendruscolo from Cambridge’s Centre for Misfolding Diseases, who led the research. “However, about a third of the proteins in our body do not fold, and instead remain in disordered shapes, sort of like noodles in a soup.”
We do not know much about the behaviour of these disordered proteins, since traditional methods tend to address the problem of determining static structures, not structures in motion. The approach developed by the researchers harnesses the power of Google's cloud computing infrastructure to generate large numbers of short trajectories. “Extensive computer simulations allow us to capture the molecular-level motions of thousands of copies of a protein in parallel, and play them back like a movie,” said co-author Dr Kai Kohlhoff from Google Research.
The most common types of motions show up multiple times in these movies, making it possible to define the frequencies by which disordered proteins jump between different states.
“By counting these motions, we can predict which states the protein occupies and how quickly it transitions between them,” said first author Thomas Löhr from Cambridge’s Yusuf Hamied Department of Chemistry.
The researchers focused their attention on the amyloid-beta peptide, a protein fragment associated with Alzheimer’s disease, which aggregates to form amyloid plaques in the brains of affected individuals. They found that amyloid-beta hops between widely different states millions of times per second without ever stopping in any particular state. This is the hallmark of disorder, and the main reason for which amyloid-beta has been deemed ‘undruggable’ so far.
“The constant motion of amyloid-beta is one of the reasons it’s been so difficult to target – it’s almost like trying to catch smoke in your hands,” said Vendruscolo.
However, by studying a variant of amyloid-beta, in which one of the amino acids is modified by oxidation, the researchers obtained a glimpse on how to make it resistant to aggregation. They found that oxidated amyloid-beta changes shape even faster than its unmodified counterpart, providing a rationale to explain the decreased tendency for aggregation of the oxidated version.
“From a chemical perspective, this modification is a minor change. But the effect on the states and transitions between them is drastic,” said Löhr.
“By making disordered proteins even more disordered, we can prevent them from self-associating in aberrant manners,” said Vendruscolo.
The approach provides a powerful tool to investigate a class of proteins with fast and disordered motions, which have remained elusive so far despite their importance in biology and medicine.
Reference:
Thomas Löhr et al. ‘A kinetic ensemble of the Alzheimer's Aβ peptide’ Nature Computational Science (2021). DOI: 10.1038/s43588-020-00003-w
The text in this work is licensed under a Creative Commons Attribution 4.0 International License. Images, including our videos, are Copyright ©University of Cambridge and licensors/contributors as identified. All rights reserved. We make our image and video content available in a number of ways – as here, on our main website under its Terms and conditions, and on a range of channels including social media that permit your use and sharing of our content under their respective Terms.