AI versus cancer
The Cambridge researchers using machine intelligence to beat disease
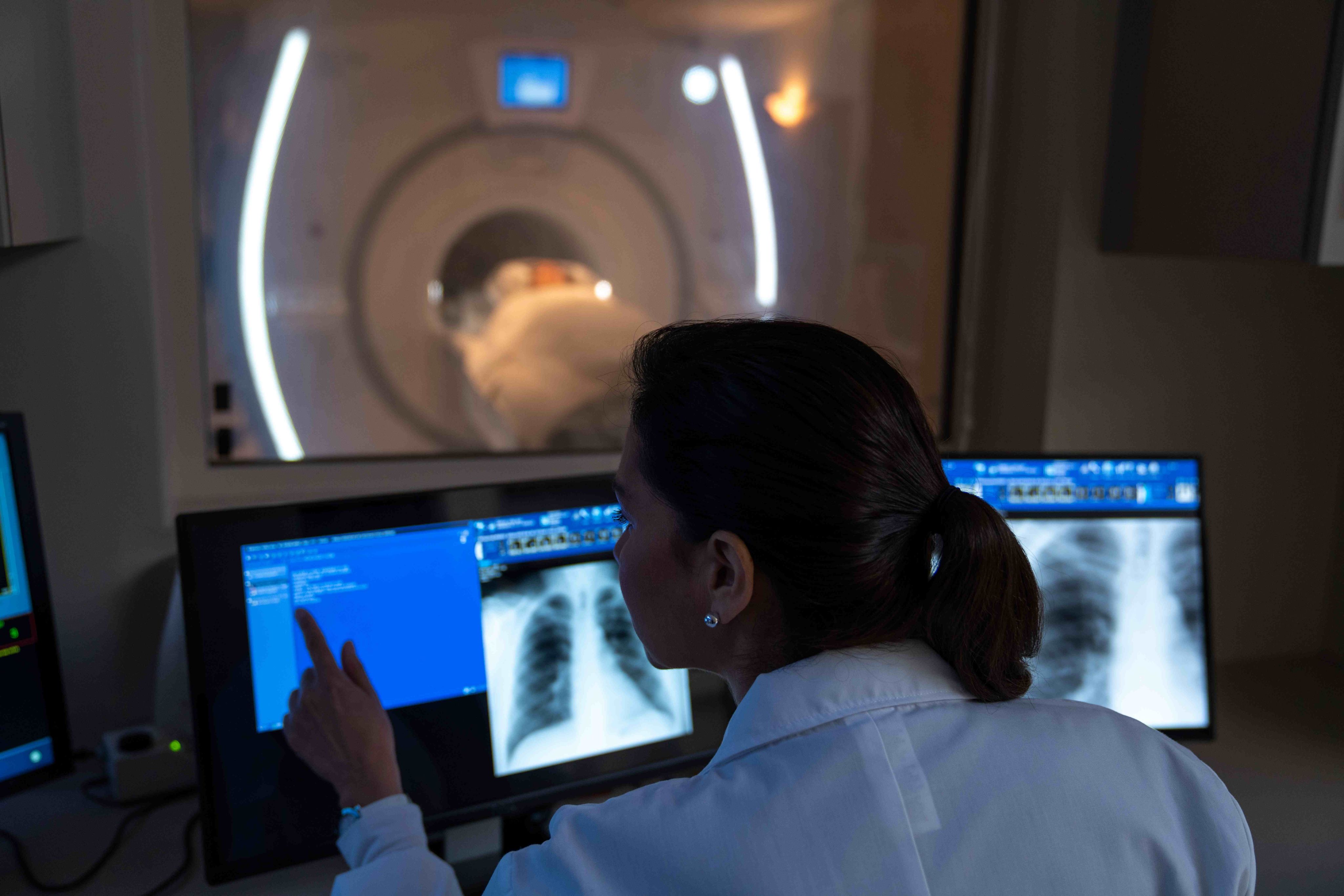
‘Game changing’ technology which can predict how patients will respond to cancer treatment before they receive it is part of a wave of Cambridge research harnessing the power of AI in the fight against the disease.
The new tool – which focuses on ovarian cancer, but has the potential to help treat other cancers – means that vital time can be saved on futile therapies and other treatments introduced sooner. The AI model uses machine learning to digitally integrate a wealth of patient data – including CT images, blood tests, genomic results, and electronic health records – to help clinicians make quicker, highly personalised, and better-informed decisions in treating ovarian cancer, which is usually diagnosed at an advanced stage when immediate intervention is key.
Most patients are given chemotherapy to shrink tumours ahead of primary surgery to remove them, however 39% of women do not respond to the chemotherapy, which only serves to delay the surgery. But using the new AI tool, IRON (Integrated Radiogenomics for Ovarian Neoadjuvant therapy), researchers were able to predict whether chemotherapy would be effective during a study involving 134 women at two centres.
Dr Mireia Crispin-Ortuzar, who along with Professor James Brenton co-leads the Cancer Research UK Cambridge Centre's Ovarian Cancer programme, said: “Essentially, it means that we can estimate who might benefit from these treatments and who might need alternatives, so we can make changes to the treatment journey sooner. It also allows us to discover reasons why patients are not responding to chemotherapy.”
Alongside clinical data and patients’ general characteristics – including age and disease status – IRON uses augmented radiomics to scan CT images, measure lesions, and reveal patterns in tumours and tissues that the human eye cannot detect, on a scale that would be prohibitively expensive to do ‘by hand'. All of this information is then analysed using the AI model to predict the likely success of chemotherapy.
“Measuring the volume of the disease is crucial in understanding and treating it,” said Dr Crispin-Ortuzar. “And to measure the disease it helps greatly if you know the boundary of the lesions. This is extremely time consuming for radiologists and takes hours out of their day. Now we have developed automatic AI tools to do this, which coupled with IRON could be game changing.”
Dr Mireia Crispin-Ortuzar
Dr Mireia Crispin-Ortuzar
Professor James Brenton
Professor James Brenton
Professor Brenton, who is also co-leader of Cambridge University’s Mark Foundation Institute for Integrated Cancer Medicine, and is based at the Cancer Research UK Cambridge Institute, said the research was hugely significant, and that AI offered clinicians “new thinking” on targeting cancer.
“AI gives us the ability to follow these structures in time and space, and to correlate the image with the genomic features,” he said. “And that is a really major step forward, and is what a complex disease like ovarian cancer really needs.
“Outcomes for patients with advanced stages of ovarian cancer haven’t changed dramatically in the past 20 years, because research hasn’t made a ‘move the needle’ difference. It needs new thinking that can sometimes be lost in more pragmatic kinds of clinical trials, which haven’t learned enough about why the cancer does or does not respond. We need completely new tools, and these AI approaches are groundbreaking in that regard.”
IRON is part of a collaboration between The University of Cambridge, Cambridge University Hospitals and GE Healthcare, which is also developing a comprehensive clinical decision-making support platform that integrates and refines cancer patient data from multiple sources into a single, much more manageable tool. It will help explain what scans or test results mean when analysed together, supporting doctors who currently receive this information in separate formats and from many different sources.
Professor Brenton said: “We believe very strongly that the only way to have enough precision to treat patients and to understand what they need requires integration of very complex data from the clinical data stage – imaging, genomes, proteins and so on – and no one's really done that at any sort of scale up until now.”
Crucially, the platform will also help doctors share expertise, technologies, and discoveries with colleagues around the country – and reach patients in other hospitals, particularly those with rarer diseases which require specialisation.
“In the short term it’s about doing a better job with the information we already have,” said Professor Brenton. “In the medium term it’s about the new discoveries that these tools will take us to – analyses of areas of the cancer that we’d otherwise have to spend huge resources and thousands of hours trying to find. And this will lead to more trials and feed into future treatment, which is extremely exciting.”
Professor Brenton with the Brenton research group
Professor Brenton with the Brenton research group
And Dr Crispin-Ortuzar said that the research was already collecting “latent” information which could point researchers in new directions.
“We can use these AI models to tell us where we should be looking next,” she said. “When we segment lesions to find where the boundaries are, we're actually having to detect all of the texture within that lesion, so we’re collecting latent information even if we’re not using it at that moment. It’s information that is hidden somewhere in the segmentation algorithm and it’s being quantified, so we can use it to see if something unique is happening. It means that these tools are helping us to create our research programmes – they’re helping us with the science and not just simply automating tasks.”
Machine learning could pave the way for kidney cancer screening programme
AI could also help doctors diagnose kidney cancer earlier, according to another new Cambridge study – by enabling cost-effective screening for those at higher risk of the disease, which kills around 5,000 people in the UK every year.
The researchers’ initial findings show that their AI model far exceeds previous attempts to automatically detect small renal cancers, and in the future could make routine health checks for the disease possible – which would otherwise be too expensive – similar to the NHS’ now-established screening programme for lung health.
Cancer Research UK Cambridge Centre-funded PhD student Bill McGough, a researcher at the Early Cancer Institute, led the study, which suggests a deep learning diagnostic tool could reach the same level of accuracy reading CT scans as radiologists, after being trained using doctors’ medical expertise.
He said: “We want to screen for cancers that might not otherwise be economically feasible to screen for, by introducing AI and using an automated, highly accurate diagnostic system that allows us to multiply radiological tasks infinitely. Our research suggests that the model we have developed could effectively replicate a radiologist’s ability to detect kidney cancer on CT scans.”
The system uses ‘convolutional neural networks’, a deep learning method for imaging analysis, to first highlight the location of kidney within a CT scan, before analysing it for the presence of cancer. It was trained on 534 patient scans, and tested on 64.
As well as reducing costs, speeding up diagnosis, and freeing up radiologists’ time, the AI tool offers safety benefits for patients – by still being able to accurately read scans which are taken using less radiation than would usually be used for diagnostic purposes, and reducing the amount of dye, or ‘contrast medium’, a patient needs to be given to show detail in the CT images.
“All of this means we have a system that could be appropriate for screening sections of the population,” said Bill. “Most people don’t notice symptoms of kidney cancer until the disease is at a more advanced stage, but now we have shown that AI could help to detect small cancers, catch the disease early, and improve survival rates.”
Cambridge PhD student Bill McGough
Cambridge PhD student Bill McGough
Dr Raj Jena
Dr Raj Jena
AI imaging uses patient digital twin to cut waiting times
AI cancer imaging application, OSAIRIS, developed by the NHS and University of Cambridge, has cut waiting times for radiotherapy for fast growing tumours by two weeks when used alongside other technologies. It means patients are beginning treatment 15 days after referral, ahead of the NHS national target of 31 days.
“We know that the earlier you start radiotherapy, the more likely you are to cure a patient,” said Dr Raj Jena, an oncologist at Cambridge University Hospitals NHS Foundation Trust, who is leading the OSAIRIS research. “So starting therapy sooner will translate into more cancer cures. No one wants to wait to start curative radiotherapy, what you want is to finish your treatment as quickly as possible.”
OSAIRIS works by significantly cutting the amount of time a doctor needs to spend drawing around healthy organs on scans before radiotherapy. Outlining the organs is critical in order to protect the healthy tissue around the cancer from radiation. It can take a doctor between 20 minutes and three hours to perform this task, per patient. This complex but routine task is ideally suited to AI with the oncologist in control, checking every scan.
Dr Jena, whose long-term collaboration with Microsoft Research on Project InnerEye helped develop OSAIRIS, said: “We take a scan of a patient and create their ‘digital twin’, which we use to prepare the radiotherapy treatment. The algorithm goes through the scan and before the oncologist even sits down to do the work, it has already marked out all the healthy structures.”
OSAIRIS is being used at Addenbrooke’s Hospital, Cambridge, in the treatment of prostate and head and neck cancers, but has the potential to work for many other types of cancer. Dr Jena says patients’ cancer journeys will be transformed as the power of AI continues to be utilised.
He said: “OSAIRIS is one tool that addresses one key step in the chain. What we now need are tools like this that speed up every step in the chain – then the whole thing gets faster. We want patients to go from the point at which they first realise that something is wrong to completing their treatment as quickly as possible.
“There is tremendous power in what we will be able to do as we get better data from healthcare settings and apply AI and machine learning techniques. The OSAIRIS algorithm was trained on about 250 patients, and it matches human performance. If you can provide 10 times that amount of data, you start to get some interesting patterns emerging, where the algorithm continues to perform well even with scans that look very different to anything it has seen before.
“The ability to take information from one problem and make use of it in another problem is of course a feature of human intelligence. This is where it gets really interesting to me, both as an AI researcher and a clinician.”
Pattern recognition tool helping researchers 'create order out of the chaos' and target deadliest cancers
Cambridge researchers have also used AI to make sense of damaged DNA to target some of the most hard-to-treat cancers – including lung, pancreatic and oesophageal cancer.
Pattern recognition tools have allowed them to ‘untangle’ DNA that has been rearranged by the disease, known as chromosomal instability (CIN), which up until now has made pinpointing drugs and therapies incredibly difficult, and has meant that treatment for some cancers has not advanced for decades.
Professor Markowetz with the Markowetz research group
Professor Markowetz with the Markowetz research group
Florian Markowetz, Professor of Computational Oncology at the University of Cambridge, who led the team, said: “These genomes are a complete mess of missing and redundant parts, and with this genomic chaos there are no good biomarkers, which means there are no good drug targets to aim treatment at. But now we can figure it out with this tool; we can find patterns in the genomes which tell us how the DNA became scrambled, which was impossible until now.”
Funded by an ERC Advanced Grant, the researchers used the tool to investigate patterns across 7,880 tumours, representing 33 types of cancer, and determined that 80% had detectable CIN. They uncovered previously hidden biomarkers in the structure of the abnormal DNA to produce a ‘compendium’ of 17 different ‘copy number signatures’ that predict how patients will respond to different drugs, and identify which drugs could help them – including drugs that have not previously been considered.
The development of the compendium led to the foundation of precision medicine start-up Tailor Bio, which is helping to bring the tool to a clinical setting, with the first clinical trials being planned.
Professor Markowetz, who is based at the Cancer Research UK Cambridge Institute, said the key step in the research and the development of the compendium – which the team is now working to extend and refine – was using AI to look at the entire landscape of the DNA, rather than just one particular part that had been altered.
“The patterns show us what caused the abnormalities in the chromosomes,” he said. “Each of these causes is a weakness in the cell, for example a DNA repair pathway that’s not working, and many of these weaknesses can be treated with drugs. We are creating order out of the chaos.”
Professor Florian Markowetz
Professor Florian Markowetz
Support cancer research at Cambridge
Cambridge hosts a cancer research community responsible for globally significant breakthroughs and innovations.
Together we are changing the way cancer is treated. Our scientists will make discoveries alongside expert clinicians treating patients, drawing on the world-leading expertise found within the University and across the Cambridge Biomedical Campus.
We will find cancer earlier and treat it better. Join us.
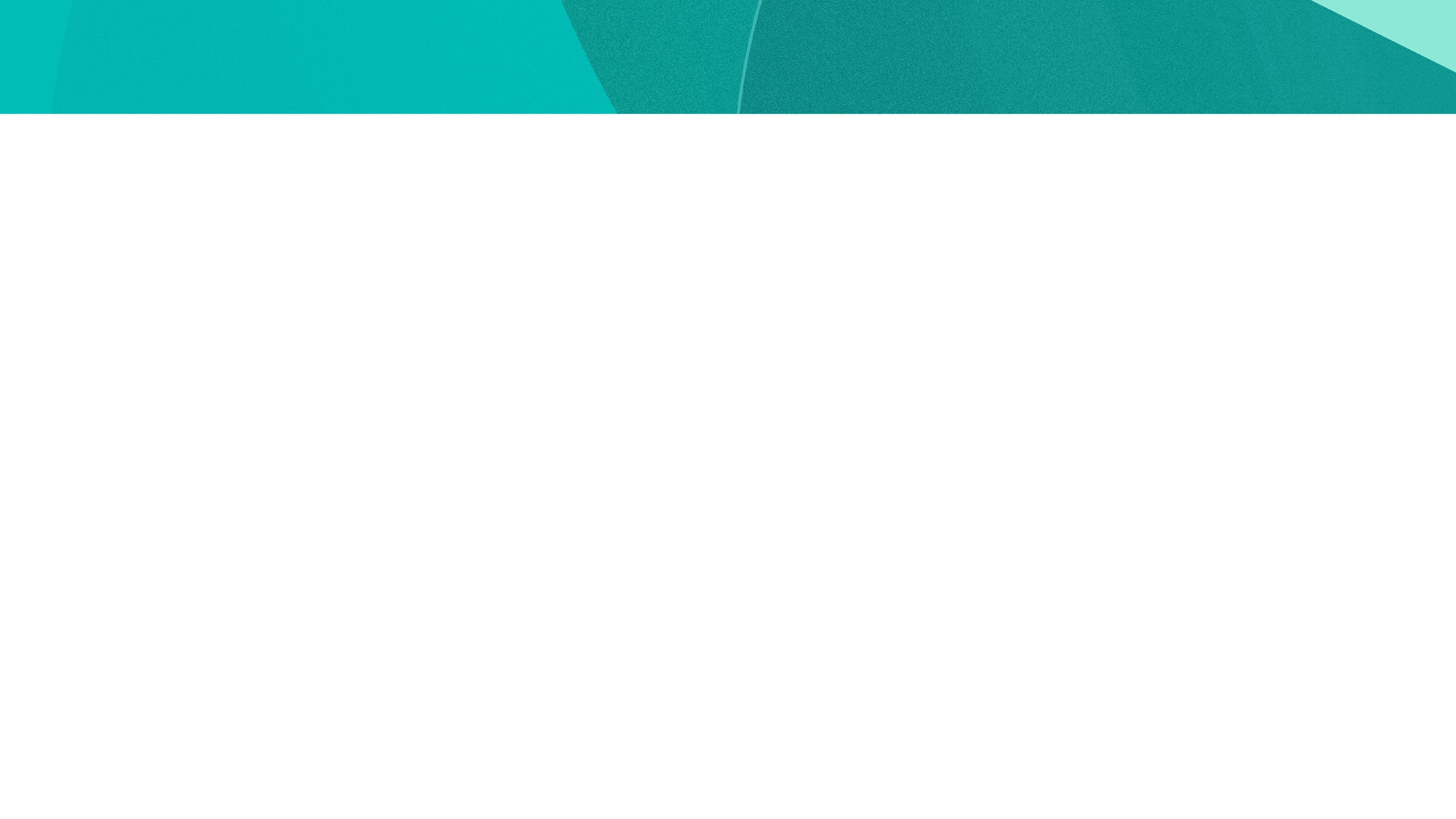
Published: 15 July 2024
Interviews and words: Stephen Bevan
The text in this work is licensed under a Creative Commons Attribution-NonCommercial-ShareAlike 4.0 International License
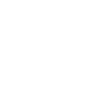