Opinion
AI can democratise weather forecasting
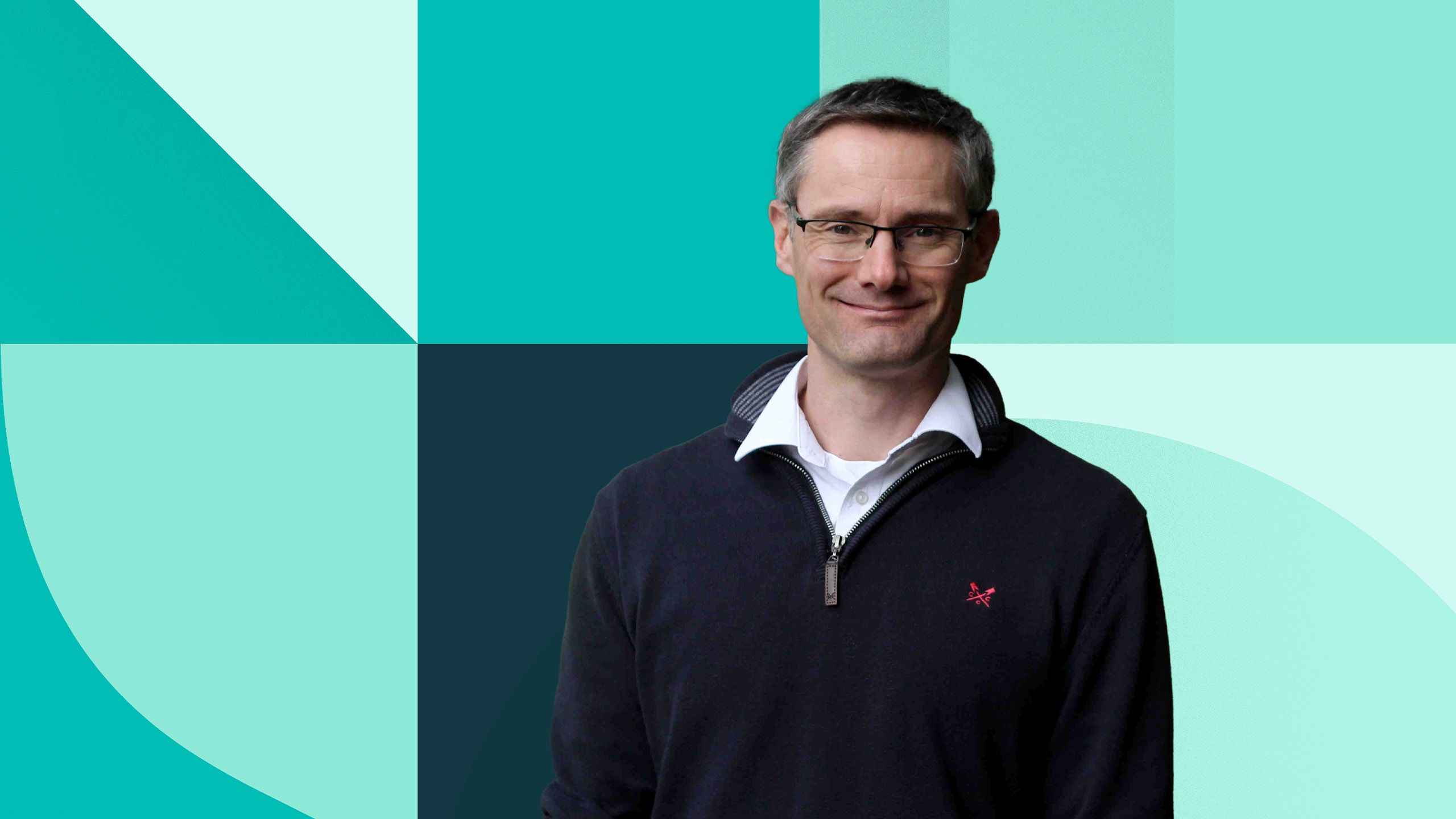
AI will give us the next leap forward in forecasting the weather, says Richard Turner, and make it available to all countries, not just those with access to high-quality data and computing resources.
From farmers planting crops to emergency responders preparing for natural disasters, the ability to predict the weather is fundamental to societies all across the globe.
The modern approach to forecasting was invented a century ago. Lewis Fry Richardson, a former student of King’s College Cambridge, who was working as an ambulance driver during the First World War, realised that being able to predict the weather could help save lives. This led him to develop the first mathematical approach to forecasting the weather.
Richardson’s method was a breakthrough, but to say that it was time-consuming is an understatement: he calculated it would require 64,000 people working with slide rules to produce a timely forecast for the following day. It was the development of supercomputers in the 1950s that made Richardson’s approach practical.
Since then, weather forecasting methods have become more sophisticated and more accurate, driven by advances in computing and by the increased amount of information we have about the weather from satellites and other instruments. But now, we are poised to make another big leap forward, thanks to AI.
The last few years have seen an AI revolution in weather forecasting and my group has recently taken this to the next level. Working with colleagues at The Alan Turing Institute, Microsoft Research and the European Centre for Medium Range Weather Forecasts, we’ve developed Aardvark Weather, a fully AI-driven weather prediction system that can deliver accurate forecasts tens of times faster and using thousands of times less computing power than both physics-based forecasting systems and previous AI-based approaches.
We believe that Aardvark could democratise access to accurate forecasts, since it can be run and trained on a regular desktop computer, not the powerful supercomputers that power most of today’s weather forecasting technology. In developing countries where access to high-quality data and computing resources is limited, platforms like Aardvark could be transformational.
AI is a game changer
The need for improved forecasting systems is more crucial than ever. Extreme weather events – from the recent wildfires in Los Angeles to last year’s flash floods in Spain – are becoming more frequent. Predicting other parts of the Earth system is equally as important. For example, 1.5 million people die each year in India due to poor air quality, and changes in ice on the sea and land at the poles have huge implications.
AI could help mitigate these risks by delivering timely, hyper-local forecasts, even in regions with limited observational data. These AI systems have the potential to dramatically improve public safety, food security, supply chain management, and energy planning in an increasingly volatile climate.
AI-driven forecasting is also well-placed to play a crucial role in our transition to a net-zero future. If we can better predict fluctuations in supply from wind and solar energy sources, we can optimise energy grids, reducing reliance on fossil fuels and making clean energy more viable on a global scale.
Richardson’s weather forecasting approach relied on numerical models – mathematical representations of the Earth’s atmosphere, land, and oceans that require massive computing power. These models, though incredibly advanced, have limitations: they are expensive, slow to run, time consuming to improve, and often struggle to deliver accurate predictions in areas like the tropics or the poles. The arrival of AI is changing the game entirely.
Achieving its potential
Results from Aardvark and other AI-driven systems have demonstrated that they can perform weather forecasting tasks with excellent speed and accuracy. These models, trained on vast amounts of historical data, can learn patterns and generate forecasts in a fraction of the time that traditional methods require. Through the Aurora project with Microsoft Research, I’ve also shown that the same approaches can transform forecasts of air quality, ocean waves, and hurricanes.
Companies like Google DeepMind, Microsoft, and various research institutions – including my team at Cambridge – are achieving results that rival or even surpass conventional numerical models at a fraction of the computational cost.
Of course, this transformation comes with challenges.
Ensuring trust and transparency in AI weather forecasting technologies is paramount. Weather forecasting has long been a domain where public institutions – like the UK Met Office and the European Centre for Medium-Range Weather Forecasts – play a critical role in ensuring reliability and accountability. AI models, though promising, must be rigorously tested and validated to build public confidence. These systems should be implemented alongside existing methods, rather than replacing them outright, and continuous retraining and re-evaluation will likely be needed due to the changing climate.
National weather services and universities like Cambridge must step up to ensure that AI-driven forecasting remains a public good, not a commercial commodity. The rise of AI weather forecasting has opened the door to more commercial involvement in an area that would previously have been dominated by public institutions and international centres. While start-ups and big tech companies are making significant strides in AI weather prediction and are a valuable part of the forecasting ecosystem, business interests are not necessarily aligned with societal need. The risk is that critical forecasting capabilities could become privatised, limiting access for those who need it most.
Universities are uniquely positioned to act as a balancing force, driving research that prioritises long-term societal benefit. However, traditional academic structures are often ill-equipped to handle the scale and speed required for AI research. If we are to compete with industry, we must rethink how AI research is conducted – embracing interdisciplinary collaboration, investing in large-scale computational infrastructure, rethinking funding models so that they are faster and more agile, and fostering partnerships that ensure AI development aligns with the public good.
The future of weather forecasting will not be decided solely in the labs of tech giants or the halls of government. It will be shaped by the choices we make now – how we invest in research, how we regulate AI deployment, and how we ensure that life-saving technology remains accessible to all.
Richard Turner is Professor of Machine Learning in the Machine Learning Group of the Department of Engineering, a Research Lead at the Alan Turing Institute, and previously a Visiting Researcher at Microsoft Research. He is also a Bye Fellow of Christ’s College.
Published 1 April 2025
Interview: Sarah Collins
The text in this work is licensed under a Creative Commons Attribution-NonCommercial-ShareAlike 4.0 International License
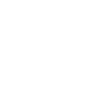