Turbocharging the race to protect NATURE and CLIMATE with AI
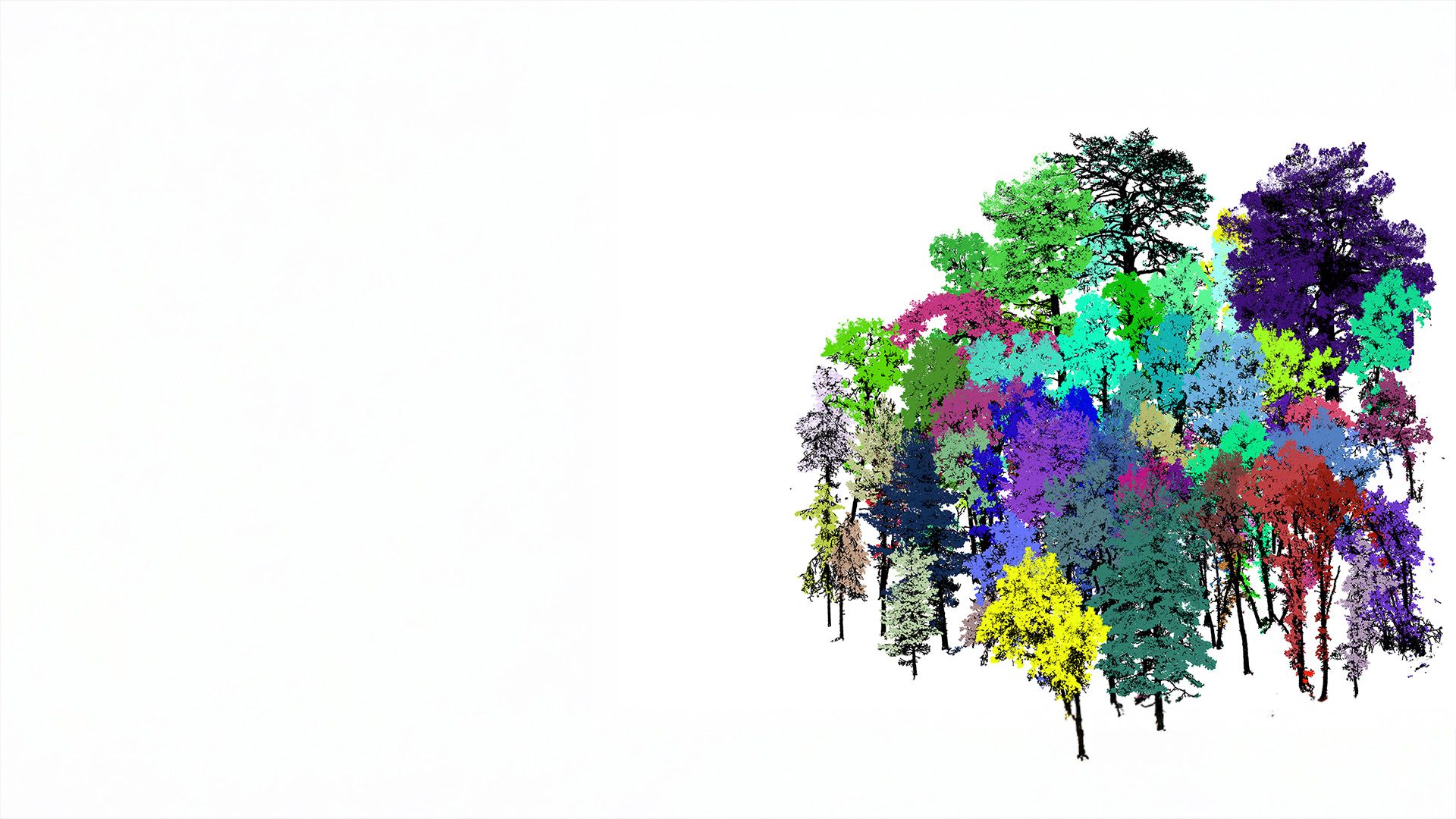
"We're in a time of unprecedented change. We must accelerate progress towards equitably rebalancing how humans and nature coexist across the world. AI is our chance to do it!"
Anil Madhavapeddy, Professor of Planetary Computing, Department of Computer Science and Technology
Across Cambridge, researchers are using AI to transform climate and biodiversity research. Find out how AI is being used in...
Improving land use decisions to benefit nature
“Around one third of the world’s land surface has been transformed for human use in the last 60 years. It’s mad just how terrible local decision-making can be for preserving global biodiversity. By protecting one place we’re often just outsourcing the impact to somewhere else,” says Anil Madhavapeddy, Professor of Planetary Computing in the Department of Computer Science and Technology.
Madhavapeddy is part of the team building a new AI tool called Terra - a predictive model of all terrestrial life on Earth. It’s a hugely complex task. The aim is to understand, then help decision-makers try to reverse, ecosystem deterioration and biodiversity loss while also producing enough food, energy and water for our needs.
Terra will combine extensive terrestrial data with earth observation data from satellites and drones, predictively filling in the blanks to build accurate global maps of biodiversity and human activity, and to reveal the world’s biodiversity hotspots.
The tool will help governments and businesses predict the global biodiversity impact of land-use decisions about vital human activities, like farming. It will vastly improve analysis of the effects of climate change, and the accuracy of biodiversity monitoring.
“Every bit of land on the planet is used for something - either by humans, or by nature. With Terra we’re trying to map it all."
"We’ll use this to ask which land is most valuable for nature, and which for humanity, on a global scale, and show the potential impact of any land-use decision - aiming to protect the highly biodiverse areas,” says Madhavapeddy.
“We’re also working with Bill Sutherland’s Conservation Evidence project, which is gathering all human knowledge of biodiversity conservation to see which interventions are most effective for each species. The Holy Grail is to combine the observational data with this knowledge-based data. Then we can build really accurate maps of the world.”
“We can’t just rewild everything or humans will starve, and we can’t expand agricultural land so everyone can eat low-intensity organic food because there won’t be enough wildlife left. Terra could help us find the solutions.”
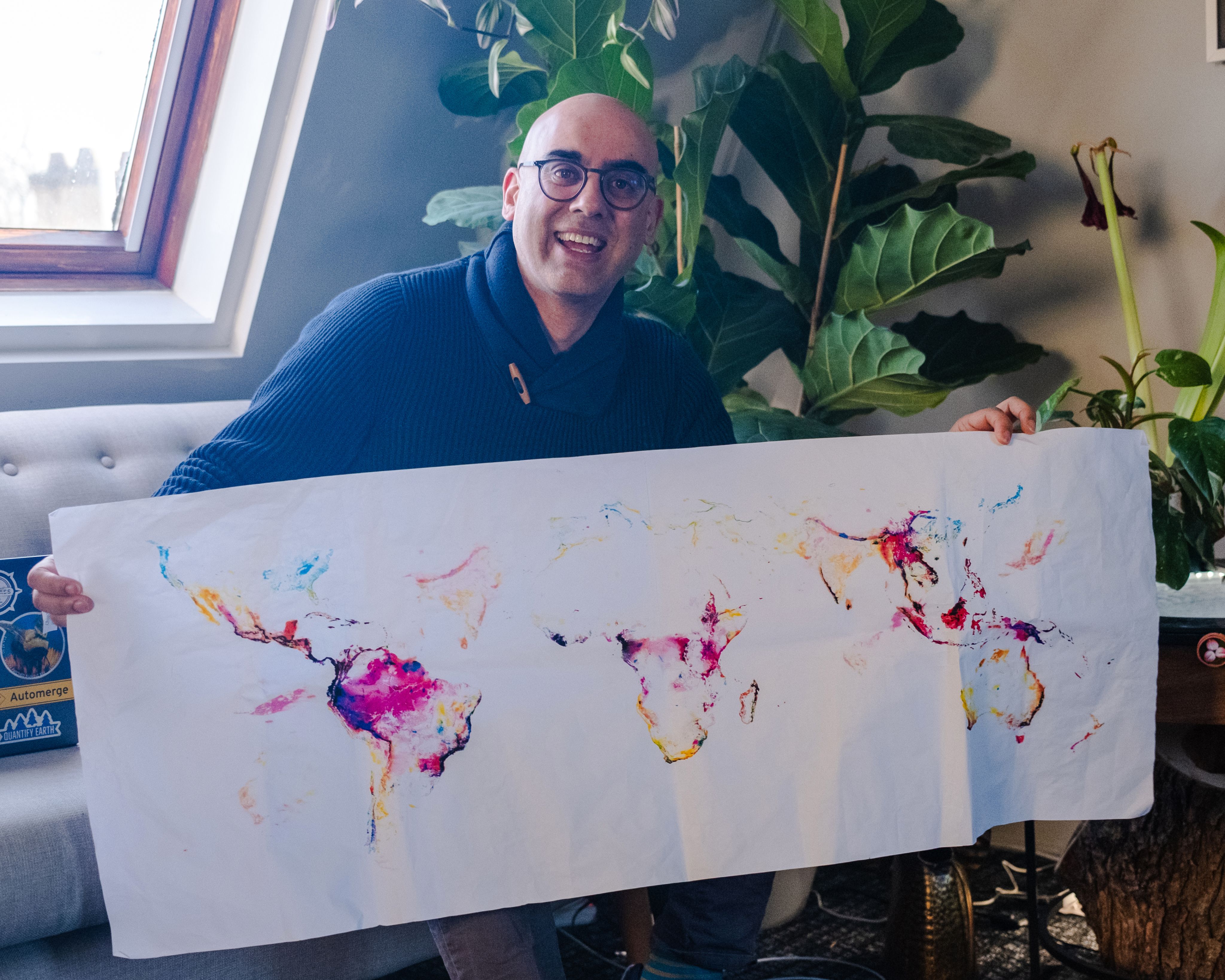
Speeding up effective biodiversity conservation
Professor Bill Sutherland has an ambitious goal: “to change the culture so it becomes unthinkable not to use evidence in conservation projects.” He wants to stop time and money being wasted on nature conservation projects that don’t work – something he’s seen surprisingly often.
Twenty years, and the equivalent of 75 years of researchers’ time, after he began the project, his freely available Conservation Evidence database is being used by a wide range of conservation organisations and policy makers, and resulting in better outcomes for nature.
But with up to one million species still facing extinction, things need to happen faster.
The team is using Large Language Models (LLMs) - a type of AI that can understand and process text, learning as it goes - to help comb through the vast conservation literature. They’re training it using their existing database, aiming for an automatic review system where AI can keep adding evidence from the ever-growing number of published papers: 300 million at last count.
"The problem is that it takes a long time to summarise evidence of what works in conservation. The team has read 1.6 million papers in 17 languages, and new science is being generated all the time," says Sutherland, Professor of Conservation Biology in the Department of Zoology, adding:
"AI can make us much more efficient. It can find papers it thinks are suitable for our database, summarise them, classify them, and explain its decisions. It’s just incredible!”
Planetary Computing Fellow Dr Sadiq Jaffer, in the Department of Computer Science and Technology, is part of the interdisciplinary team building a ‘Conservation Co-Pilot’ based on the system. This will guide decision-makers towards the best way to manage specific land types to conserve particular species - and should be available within a year.
“The Conservation Co-Pilot will enable people to get answers to specific questions using the Conservation Evidence database almost instantaneously – quite a contrast to a traditional systematic review, which might take a year and cost £100,000,” says Jaffer. “Humans will still make the decisions, but the Co-Pilot will suggest the best course of action for nature, and can massively increase productivity."
More: A man with a big idea. AI-Driven Conservation Co-Pilot is supported by ai@cam.
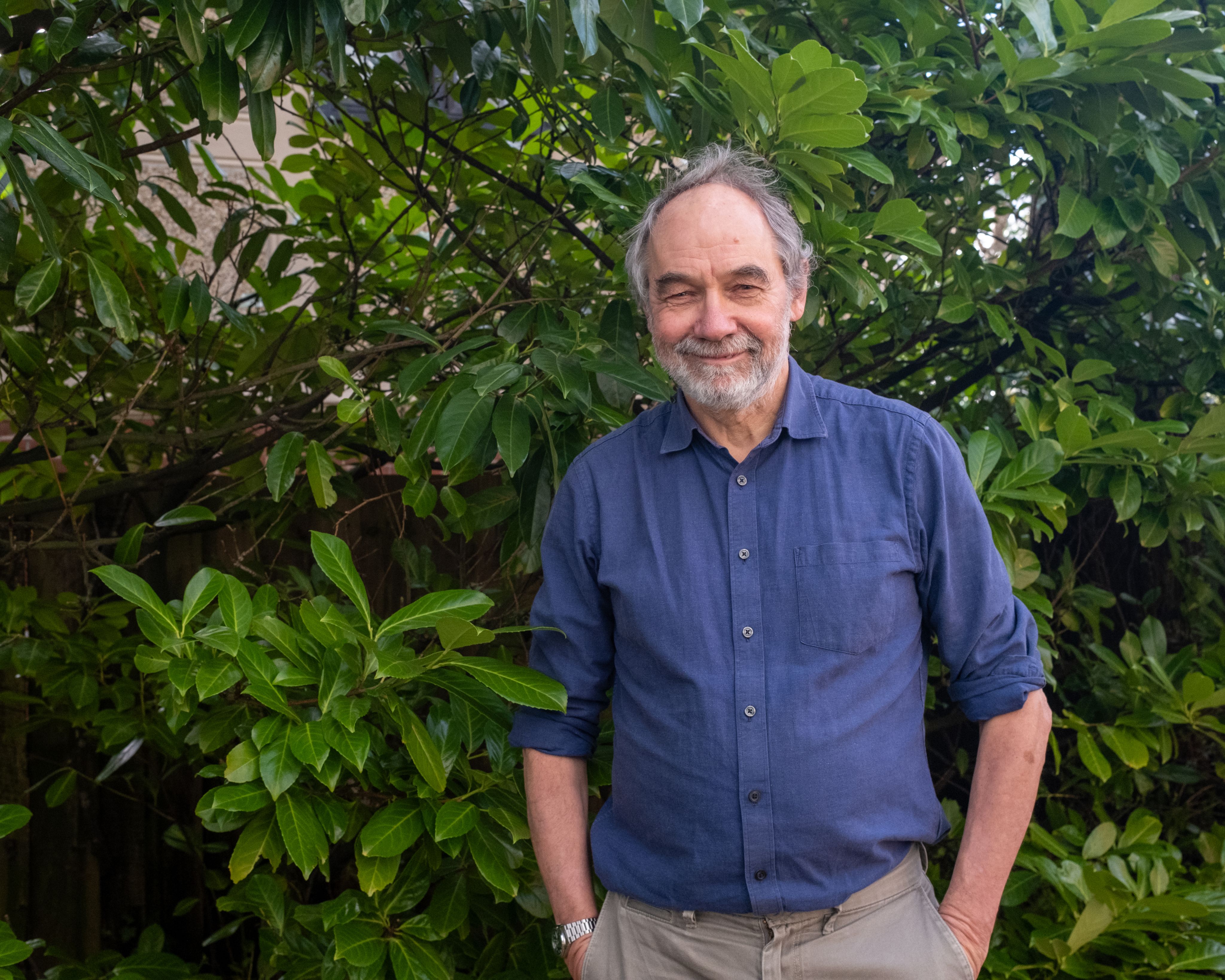
Understanding climate complexity for better forecasting
If hurricane warnings were taken more seriously because they were highly accurate, could more lives be saved? If we’d foreseen the current state of our climate fifty years ago, could more have been done to curb global temperature rise?
As the climate warms, the Earth’s natural systems are starting to behave in increasingly unpredictable ways. The models behind both short and long-term climate forecasts are getting more complex, and huge amounts of data being gathered, as scientists scramble to work out what’s going on. Machine learning, and software engineers, are becoming vital.
“Reliable forecasts of future climate trends - like temperature rise and sea-level change - are crucial for policy-makers trying to plan for the impacts of climate change,” says Dr Joe Wallwork, a Research Software Engineer at Cambridge’s Institute of Computing for Climate Science (ICCS), adding:
“We need better early warning systems to accurately forecast when and where extreme events will occur.”
“A lot of climate models are limited in resolution,” adds Dr Jack Atkinson, also a Research Software Engineer at ICCS. “Climate processes can happen at very small scales, and machine learning can help us improve the representation of these processes in models.”
Atkinson is lead developer of FTorch, a software that bridges the gap between traditional climate models and the latest machine learning tools. This breakthrough is improving climate predictions by better representing small-scale processes that are challenging to capture in models. FTorch is now used by major institutions, including the National Center for Atmospheric Research, to enhance climate simulations and inform global policy.
Models in development by the team, which use FTorch, use fewer assumptions and more real climate data. They’re powering the science of many climate studies - from sea-ice change, to cloud behaviour, to greenhouse gases in the atmosphere.
They’re helping to make climate predictions faster, which has important climate implications too. “It’s an unfortunate irony that climate models require quite a bit of energy to run,” says Wallwork. “With machine learning we can make the models more efficient, running faster so we can potentially lower our emissions.”
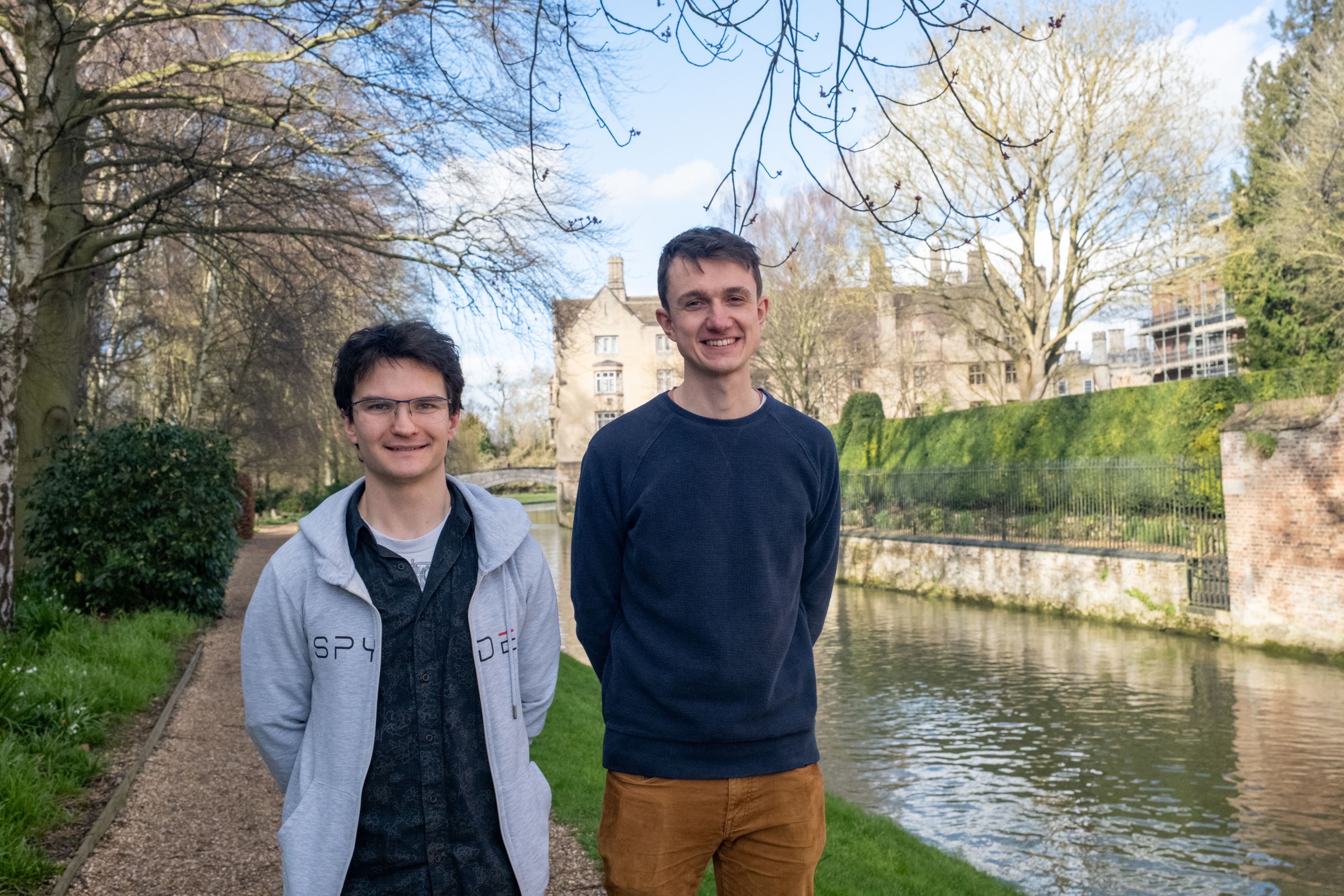
Towards more energy-efficient homes
Dr Ronita Bardhan, Associate Professor of Sustainable Built Environment, Department of Architecture and her team have developed an algorithm using open-source data, that uses satellite images to reveal the heat loss from almost every home in the UK.
This is helping identify the homes at risk of losing most heat, which could be prioritised to improve their energy performance. Bardhan has identified almost 700 homes in Cambridge alone that are particularly vulnerable to heat loss and hard to decarbonise.
The UK government is currently consulting on whether private rental homes should meet a minimum energy efficiency standard (an EPC rating of C) – with the outcome due in May 2025. A limited number of homes have an EPC rating, but according to Bardhan’s research, over half of the houses in England and Wales would fail this requirement.
“Our aim is to inform policy discussions in support of decarbonisation strategies. We’re using AI to unearth the hidden layers affecting population health, including the energy efficiency of our homes.”
Bardhan adds: “Prioritising the risks faced by individual households must become a central focus in climate change discussions. By capturing thermal images, we can distinctly visualise rooftops, walls, and other structural elements to precisely identify how each building loses heat."
“This allows us to ask critical questions: How much of this heat could be retained through retrofitting? How can we empower households to become more resilient in the face of a changing climate? It allows us to identify poorly performing homes, and those whose owners most need government support to increase the property’s energy efficiency. To reach net zero by 2050 this must be done as a priority.”
Household heat loss isn’t the only issue that can affect health – indoor overheating can too. In the summer of 2022, thousands of people died in the UK due to extreme heat.
“We want to take maximum advantage of our algorithm – scaling it up to look not only at heat loss from homes for the winter months, but also what happens during the summer months when outside temperatures rise,” she says, adding:
“As the climate warms, buildings need to become more energy-efficient to help combat climate change and avoid excessive energy consumption. We shouldn’t need to use air conditioning in the UK!"
More: https://www.sustainabledesign.arct.cam.ac.uk/projects/decarbonisation. Bardhan's research is supported by the UK and European Space Agencies. She contributes to the Health-Driven Design for Cities (HD4) project based at CARES in Singapore.
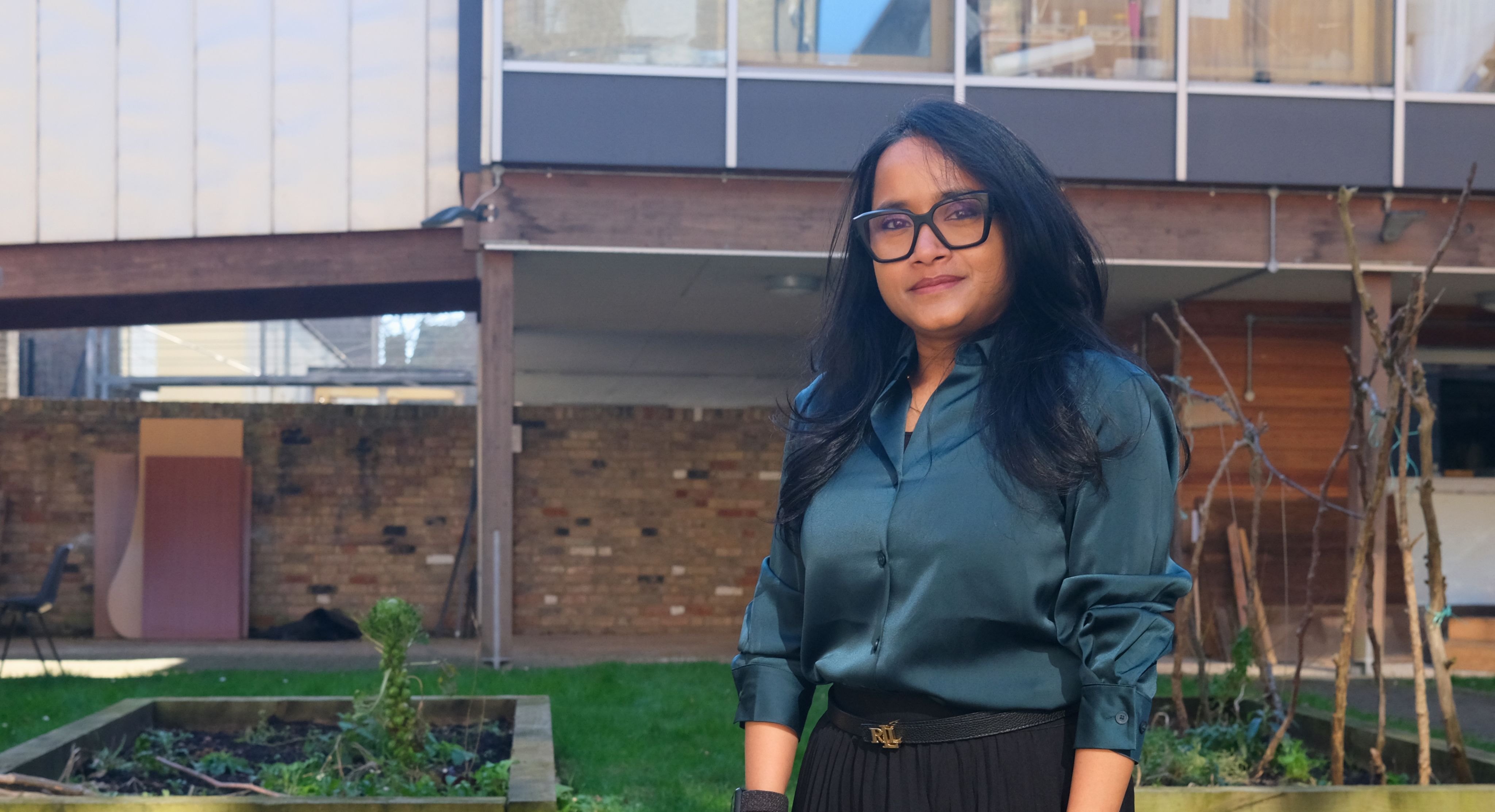
Enhancing forest monitoring and carbon tracking
The immense value of forests for capturing atmospheric carbon and supporting biodiversity is now clear. As market initiatives like carbon and biodiversity credits gain momentum to help offset our environmental impact, the science behind them is evolving too. Two new Cambridge projects are harnessing the power of AI to improve forest monitoring and carbon tracking, by diving into remarkable levels of detail.
“Our AI model will provide stronger evidence to support programmes for carbon accounting and biodiversity credits, by validating large-scale satellite images with extensive, high-quality data collected on the ground,” says Dr Emily Lines in the Department of Geography.
She’s working with Dr Harry Owen, also in the Department of Geography, to develop an AI model trained on data collected across European forests. This includes billions of terrestrial laser scans, drone images, and even manual measurements with a tape. While the sheer volume of data has previously been difficult to manage, AI is now speeding up the process and making the data easier to interpret.
“AI allows us to create high-resolution, high-quality and large-scale estimates of ecosystem properties – including their species composition and ecological function. This opens up new opportunities to rigorously test and validate credit-based valuations of ecosystems,” says Lines.
In a complementary project, researcher Frank Feng in the Department of Computer Science and Technology has developed an app called GreenLens, to measure trees on the ground much more quickly and accurately than a person with a tape measure. Estimating tree trunk diameter is key in understanding a tree’s health and its ability to store carbon. The freely available app is a user-friendly tool that provides reliable data to support reforestation projects and advance sustainability efforts.
“GreenLens uses AI-powered computer vision and depth detection to measure tree trunk diameters on affordable Android devices. This makes it easier and faster for researchers, landowners, and communities to collect data and monitor forests - without the need for expensive equipment,” says Feng.
Together these projects demonstrate the growing role of AI and mobile technology in improving forest monitoring, accelerating effective climate action, and supporting biodiversity conservation worldwide.
More: Harnessing AI for Forest Monitoring is supported by ai@cam.
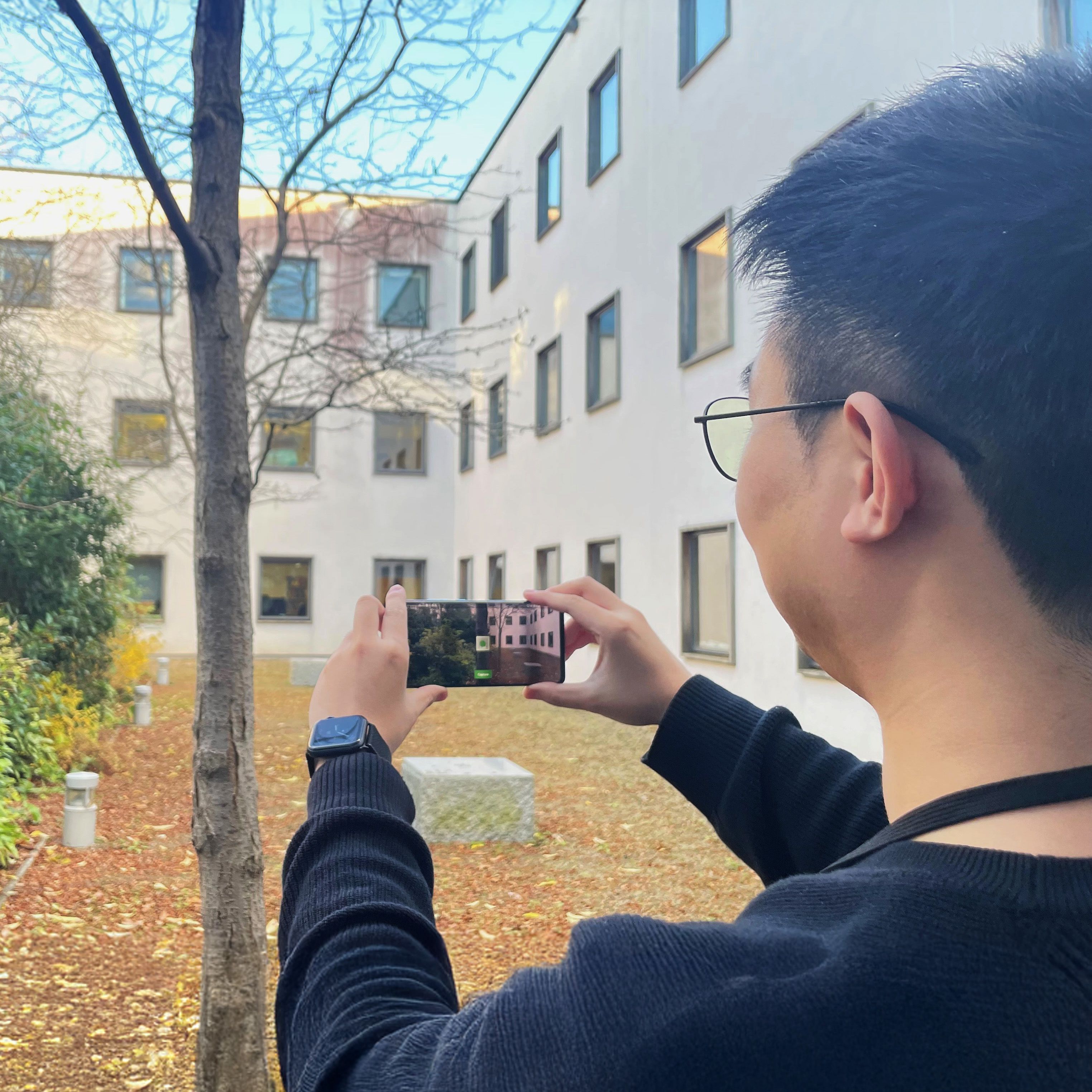
AI can help heal
us heal the planet
Professor Anil Madhavapeddy on how the speed of AI is why we must harness it to reverse the damage we’ve done to the planet. Read more
Published 6 April 2025
Images (Top): 3D visualisation of a Spanish forest plot captured with Terrestrial Laser Scanning. Colours highlight individual trees, while AI-powered wood classification is shown in black. Credit Harry Owen. (Bottom) Frank Feng, credit Rachel Gardner. All other photographs by Jacqueline Garget.
The text in this work is licensed under a Creative Commons Attribution-NonCommercial-ShareAlike 4.0 International License
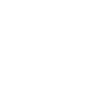