Opinion
AI can transform health
and medicine
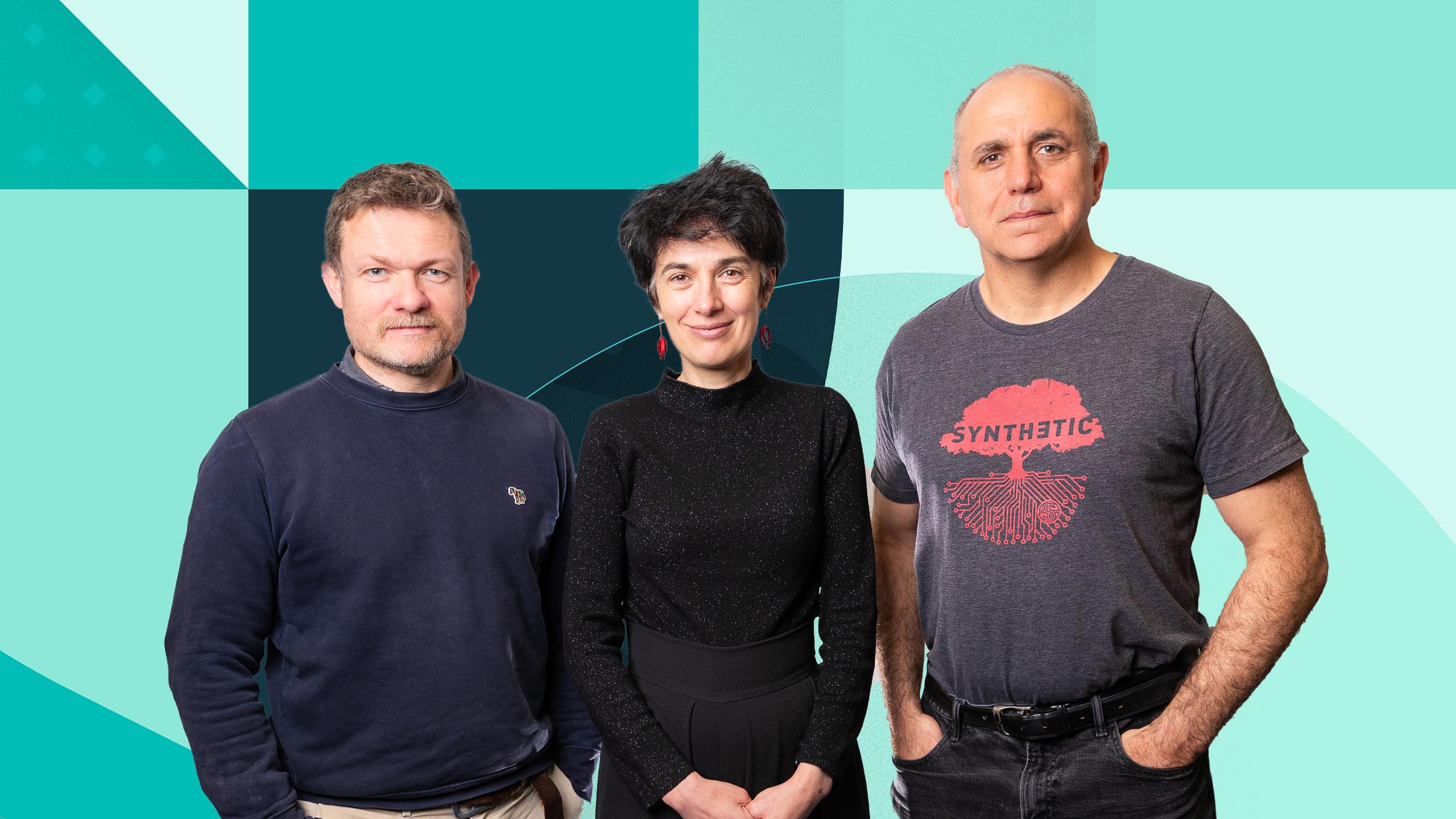
AI has the potential to transform health and medicine. It won't be straightforward, but if we get it right, the benefits could be enormous. Andres Floto, Mihaela van der Schaar and Eoin McKinney explain.
If you walk around Addenbrooke’s Hospital on the Cambridge Biomedical Campus, sooner or later you will come across a poster showing a clinician in his scrubs standing by a CT scanner, smiling out at you.
This is Raj Jena, one of our colleagues and Cambridge’s first – in fact, the UK’s first – Professor of AI in Radiology. One of the reasons Raj has been chosen as a face of Addenbrooke’s is his pioneering use of AI in preparing radiotherapy scans. OSAIRIS, the tool he developed, can automate a complex, but routine task, saving hours of doctors’ time and ensuring patients receive crucial treatment sooner.
It’s just one example of the ways that AI will transform medicine and healthcare – and of how Cambridge is leading the charge.
The impact of AI in medicine will likely be in four main areas:
First, it will ‘turbocharge’ biomedical discovery, helping us to understand how cells work, how diseases arise, and how to identify new drug targets and design new medicines.
Second, it will unlock huge datasets – so-called ‘omics’, such as genomics and proteomics – to help us predict an individual’s disease risk, detect diseases early and develop more targeted treatments.
Third, it will optimise the next generation of clinical trials, allowing us to recruit the most suitable participants, and analysing and interpreting outcomes in real time so that we can adapt the trials as they go along.
All of these will lead to the fourth way – transforming the treatments we receive and the healthcare systems that deliver them. It will allow us to personalise therapies, offering the right drug at the right time at the right dose for the right person.
Achieving its potential
None of this, of course, will be straightforward.
While the technical knowhow to develop AI tools has progressed at almost breakneck speed, accessing the data to train these models can present a challenge. We risk being overwhelmed by the ‘three Vs’ of data – its volume, variety and velocity. At present, we’re not using this data at anywhere near its full potential.
To become a world leader in driving AI innovation in healthcare, we will need massive investment from the UK government to enable researchers to access well-curated data sets. A good example of this is UK Biobank, which took a huge amount of foresight, effort and money to set up, but is now used widely to drive innovation by the medical research community and by industry.
Clinical data is, by its very nature, highly sensitive, so it needs to be held securely, and if researchers want to access it, they must go through a strict approvals process. Cambridge University Hospitals NHS Foundation Trust has established the Electronic Patient Record Research and Innovation (ERIN), a secure environment created for just this reason, with an audit trail so that it is clear how and where data is being used, and with data anonymised so that patients cannot be identified. It is working with other partners in the East of England to create a regional version of this database.
We need this to happen at a UK-wide level. The UK is fortunate in that it has a single healthcare system, the NHS, accessible to all and free of charge at the point of use. What it lacks is a single computer infrastructure. Ideally, all hospitals in the UK would be on the same system, linked up so that researchers can extract data across the network without having to seek permission from every NHS trust.
Of course, AI tools are only ever going to be as good as the data they are trained on, and we have to be careful not to inadvertently exacerbate the very health inequalities we are trying to solve. Most data collected in medical research is from Western – predominantly Caucasian – populations. An AI tool trained on these data sets may not work as effectively at diagnosing disease in, say, a South Asian population, which is at a comparatively higher risk of diseases such as type 2 diabetes, heart disease and stroke.
There is also a risk that AI tools that work brilliantly in the lab fail when transferred to the NHS. That’s why it’s essential that the people developing these tools work from the outset with the end users – the clinicians, healthcare workers, patients, for example – to ensure the devices have the desired benefit. Otherwise, they risk ending up in the ‘boneyard of algorithms’.
Public trust and confidence that AI tools are safe is a fundamental requirement for what we do. Without it, AI’s potential will be lost. However, regulators are struggling to keep up with the pace of change. Clinicians can – and must – play a role in this. This will involve training them to read and appraise algorithms, in much the same way they do with clinical evidence. Giving them a better understanding of how the algorithms are developed, how accuracy and performance are reported and tested, will help them judge whether they work as intended.
Jena’s OSAIRIS tool was developed in tandem with Microsoft Research, but he is an NHS radiologist who understood firsthand what was needed. It was, in a sense, a device developed by the NHS, in the NHS, for the NHS. While this is not always essential, the healthcare provider needs to be involved at an early stage, because otherwise the person developing it risks building it in such a way that it is essentially unusable.
Speaking each other’s language
In 2020, Cambridge established a Centre for AI in Medicine with the ambition of developing ‘novel AI and machine learning technologies to revolutionise biomedical science, medicine and healthcare’.
The Centre was initially set up with funding from AstraZeneca and GSK to support PhD studentships, with each student having as supervisors someone from industry, a data scientist and a ‘domain expert’ (for example, a clinician, biologist or chemist). Another industry partner – Boehringer Ingelheim – has since joined.
We are very fortunate in Cambridge because we have a mixture of world-leading experts in AI and machine learning, discovery biology, and chemistry, as well as scientifically-minded clinicians who are keen to engage, and high performance computing infrastructure, such as the Dawn supercomputer. It puts us in the perfect position to be leaders in the field of AI and medicine.
But these disciplines have different goals and requirements, different ways of working and thinking. It’s our role at the Centre to bring them together and help them learn to speak each other's language. We are forging the road ahead, and it is hugely exciting.
If we get things right, the possibilities for AI to transform health and medicine are endless. It can be of massive public benefit. But more than that, it has to be.
Professor Andres Floto and Professor Mihaela van der Schaar are Co-Directors of the Cambridge Centre for AI in Medicine. Professor Eoin McKinney is a Faculty Member
Published 7 April 2025
The text in this work is licensed under a Creative Commons Attribution-NonCommercial-ShareAlike 4.0 International License
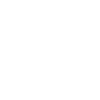